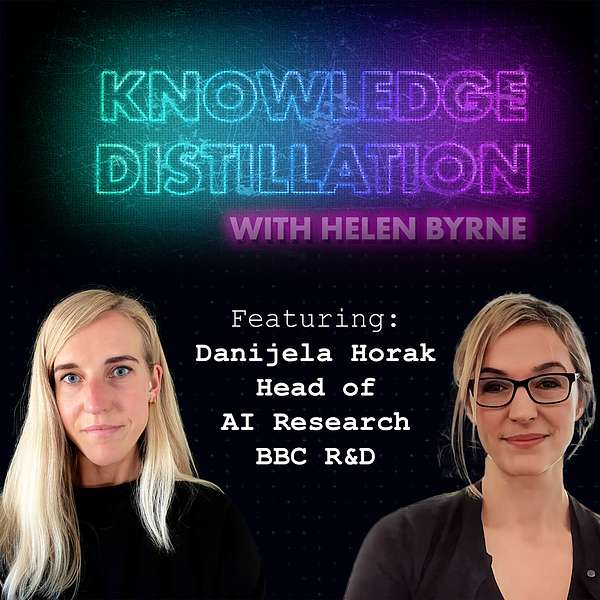
Knowledge Distillation with Helen Byrne
Knowledge Distillation is the podcast that brings together a mixture of experts from across the Artificial Intelligence community.
We talk to the world’s leading researchers about their experiences developing cutting-edge models as well as the technologists taking AI tools out of the lab and turning them into commercial products and services.
Knowledge Distillation also takes a critical look at the impact of artificial intelligence on society – opting for expert analysis instead of hysterical headlines.
We are committed to featuring at least 50% female voices on the podcast – elevating the many brilliant women working in AI.
Host Helen Byrne is a VP at the British AI compute systems maker Graphcore where she leads the Solution Architects team, helping innovators build their AI solutions using Graphcore’s technology.
Helen previously led AI Field Engineering and worked in AI Research, tackling problems in distributed machine learning.
Before landing in Artificial Intelligence, Helen worked in FinTech, and as a secondary school teacher. Her background is in mathematics and she has a MSc in Artificial Intelligence.
Knowledge Distillation is produced by Iain Mackenzie.
Knowledge Distillation with Helen Byrne
Danijela Horak - Head of AI Research, BBC R&D
Danijela Horak explains how the BBC is making use of AI and its plans for the future, including detecting deepfakes as well as using deepfake technology as part of its production process.
Danijela and Helen discuss the Corporation's use of open source models and its view on closed source technologies such as the GPT family of models from OpenAI.
We find out how the BBC uses AI for recommendation, while taking cautious approach to user data, and Helen and Danjela reflect on why there needs to be more rigour in AI research so that the field doesn't end up on a par with 'social sciences'!
Watch the video of our conversation with Danijela at: https://www.youtube.com/watch?v=QOwecs8KRLg
Helen Byrne
Hello, and welcome to the Knowledge Distillation podcast with me Helen Byrne. In this episode, we're joined by Danijela Horak, who leads AI research at the BBC. Danijela tells us about the ways in which they're using AI, including transcribing the entire BBC archive, and about how she would like to see a bit more scientific rigor in the AI research space. I hope you enjoy our conversation. Hi, Danijela, thank you so much for joining us on the Knowledge Distillation Podcast. How are you doing?
Danijela Horak
I'm fine. How are you Helen?
Helen Byrne
I'm good. I'm good. Thank you. So I've been lucky to kind of meet you and interact and run into you at a number of AI events in London. And I'd love sort of to set the scene for everyone else, if you could tell us a bit of a brief background on your bio and what you've done leading up to and including your role now at the BBC.
Danijela Horak
Yeah, well, at the moment, I'm a head of AI research in R&D at the BBC. And I wish I could say that my journey was like a straight line, my career journey, it was more like a Brownian motion. I started in the field of pure maths, I've done a PhD in computational algebraic topology, and then did have two postdocs, gradually moving away from pure mathematics. And then ended up with a kind of a short stint in a startup. This is almost like a rite of passage for everyone. And I spent the chunk of my ML career the major, like the biggest part in AIG, we had an AI team there, investments AI, that was our name, and everything I know, I've learned there. I mean, in terms of machine learning, and then last year, summer I moved to the BBC.
Helen Byrne
I'd love to hear I guess about all the exciting things and ways that you are using AI at the BBC. I'm sure there's a huge plethora of applications there. Maybe to set the scene a bit, could you could you tell us about what your remit looks like? So you're leading research AI? I'm sure that's slightly different to actually kind of deploying AI in different areas. So what is your remit? Like in terms of how you are bringing AI to the BBC?
Danijela Horak
So at the BBC, just going like one step back, we have three AI ML data teams. So one is in R&D. We're doing research, and I'll tell you a little bit about like what we do afterwards. Then there is a data science team in product. So they are there to serve products, they are mainly dealing with the recommenders and the metadata. And then there is a data science team in audiences, so they are in particular looking at the audience data and doing a mix between data analytics and data science. In our team, we are primarily looking at the natural modalities of data. So not like a typical data analyst data science type of work. So and we're dealing with the state of the art models, so looking whether we can utilize the new models, new technology that that is, you know, very recently published to some of the use cases that we have in the BBC. So we have like three verticals in my team, computer vision. So in computer vision, they are looking at restoration and colorization of the old archive footage. Most recently now we've started looking at some applications in AI safety. So looking at whether we can develop a watermark for the BBC video and image assets, visual assets, we're looking at the detection of deep fakes. And we're looking at the anonymization of using deep learning of people in some video programs. So you can imagine there are video programs that the BBC is shooting that may require people to be anonymized, like programs where we have witnesses and whatnot. And at the moment, we're actually researching whether this could be done at the production grade level.
Helen Byrne
Could you see, just thinking aloud, could you see how this could develop in the future to a place where you don't even need the actor. Sorry to all the actors out there worrying about losing work. Because because you can actually just generate an AI generated replacement for the for the anonymous, the person that...
Danijela Horak
Like Synthesia is doing? Yeah. Yeah, I think, I don't know, obviously, they are doing it already. We are not in that business that I think, you know, I think also, at the moment, it's quite difficult to kind of capture all of the micro movements in the synthetic avatars. So they do still look a little bit robotic. But, you know, we're looking at this very, very, very narrow domain, such that it's more kind of visually pleasing for the audience to see a face instead of kind of a blurred image, we're not kind of considering kind of general more broader use cases. So that's the computer vision, then we have some activities in seeing speech to text that I'm super excited about. So obviously, when Whisper came out, we had a very long history of looking at the speech to text. So back in 2016, to 2017, we transcribed the whole archive using the old systems, Kaldi-based systems. And now we've started experimenting with Whisper and fine tuning it on British accents. So we've got kind of squeezed out a little bit of performance from that work, and then we are going to be soon deploying it.
Helen Byrne
Wow. I keep seeing Whisper, distilled Whisper, faster Whisper. All of these improved, more efficient versions of Whisper that are coming out? Is that important for you, for your use case to keep trying to eke out as much efficiency from these models? Or do you focus more on the accuracy or both?
Danijela Horak
Absolutely. I think the primary reason that we've done a Kaldi speech-to-text text to begin with, is because we needed to transcribe the whole archive, and it would have been millions in AWS like to use commercial grade systems. So it's like thousands, hundreds of thousands of hours of audio that we need to transcribe. So every percentage for us is that is a big, big, big win.
Helen Byrne
Did you see the Whisper v3, that OpenAI dropped for their dev day, just last week, they've they've updated, the Whisper Large to a v3 version, which is meant to be much, much higher accuracy. And yeah,
Danijela Horak
Yeah, well Whisper 2 was it was pretty good to begin with. We've started looking at it and benchmarking it, how it performs. We were like, we actually started very early on that journey with Whisper integrated a lot of features like speaker diarization, we fixed the timestamps and whatnot, only to have OpenAI release or have another release later on and fix all of these issues. So I think this applies generally not only like for speech-to-text, like Whisper technology, I think it's the same with, like, when you're trying to build NLP products, like you always start with something, and it's the easiest thing to do is to start with a low hanging fruit, right. But if you do that, then you're at a huge risk of having, you know, made your work unnecessary with the next release of with the next version of the GPT, or their next release of features or whatnot. So it's a kind of a very difficult space to navigate for us, you know, for the people who are working in the field, but not there, like in the top tech firms. It's very difficult to say, well, you know, what do I build now?
Helen Byrne
So that's vision, speech. And then I guess, NLP, NLP, yeah. Text? Yeah. Do you to split into three teams like that in different modalities.
Danijela Horak
Yeah, we tried to do, although we were like a small team, we try to organize ourselves in a very modern way. So like, we have these verticals. And then when you have a project, sometimes this project kind of encompasses many verticals. So like you need people sometime. So for example, the project that we're working on at the moment, takes some people from computer vision, NLP and the engineering verticals. So we try do do that. We've been looking at building some PDF parser PowerPoint parser. So we needed a way to extract some of the artifacts from from this documents in a more clever way than...
Helen Byrne
Interesting. So another thing that I recall from the OpenAI dev day was they've just announced they also have integrated a PDF parser with ChatGPT. So it makes it really easy to use. So a lot of the organizations that I speak to, they actually are not allowed to use these APIs from companies like OpenAI, because they're have security restrictions on sending their data to third parties. So what's that like for you at the BBC? Do you have restrictions on using these? And does that mean, you have to use more open source models?
Speaker 1
Yeah. Well, that's, that's actually a very good question. So we've spent like, probably six to eight months waiting for to get approval to use API's of OpenAI for research purposes, I think because it's so new, and especially for the organizations that are super risk averse, and super worried. Like, you have to jump through through many, many, many, many hoops and involve many people, before you get the approvals even for the like, almost very low risk use cases. And because you're the first one, like you're paving that way for for everyone else in the organization, it is really a very difficult and a long process. Yeah, yeah, it's definitely not easy. Obviously, open source has its own other issues, I would say, you know, the commercial APIs are good in that they provide a better safety guardrails. Whereas, you know, with the things like a Llama or, you know, MPTs, or Mistral, you just don't have that.
Helen Byrne
So if you're trying to use open source models, do you have to kind of constantly keep up with them? When a new one comes out? Someone tests it, do you, see what how it works with your data, obviously, you can't tell immediately, once it's released, actually how well, it will perform with your use case with your data. So do try and keep on top of that?
Speaker 1
Well, I mean, not like, we're not kind of tracking the Hugging Face leaderboards every day, obviously, there are a couple of models out there and a lot of variations. So we would normally, you know, a lot of big ones would would, we would... We've played around or tested Falcon, which wasn't wasn't very good for our use case, we've looked at Llama. And that's about it, you know. Mistral... obviously, you know, it was released recently, I guess. And I thought that it was quite interesting approach, in a sense, like, they kind of posit that it's not only like, with the scaling laws, it's not only like, the compute where you like, for a fixed amount of compute, you have to optimize for the number of tokens and the size of the model, it's also the inference. So if like, you're kind of building a model, everyone else is going to use later on, maybe you should train it for a bit while longer to squeeze out the performance to then later on, like, have a better inference quality, and, you know, with a smaller model, you can achieve so much more. So definitely, this is something and this is I think the Mosaic guys also have kind of also noticed as well. So, I mean, definitely, we're, we're still at the very beginning of this journey, and are exploring our options, basically. But I thought, you know, going back to, you know, use of commercial APIs and integrating them in product, I thought that was an interesting anecdote like that people nowadays are primarily kind of integrating these commercial APIs. And, you know, if you use a maybe not GPT3.5, but GPT4, you know, you're basically surfacing it as a free service somewhere, and someone can just come and do their homework on your kind of customer service chatbots or write an essay or, you know, for other other purposes, basically. So it's very difficult, like, because you're using, because for these purposes, I would imagine that, you know, using a smaller model that is dumber, would be better than, you know, using a very powerful model and then having having to build on top of it, like all of these guardrails that someone doesn't, doesn't really misuse it. So, so definitely, you know, we will be looking at these smaller models, from the point of view of like, well, you know, there are simply use cases for which it's easier to use a dumber model, and maybe even safer, you know.
Helen Byrne
Yeah, yeah. Yeah, that's a good point. I think on the size of the model. I feel like for a while for until fairly recently, we were just getting bigger and bigger and bigger. And there was just a very clear kind of push that to scale. And it worked very well. But I have noticed just in the last three, six months, more and more hearing about models, they're sticking to the seven or the 30. The size models, obviously, the GPT 3.5 Turbo. In the Microsoft paper a few weeks ago, they released it was 20. Was it 20 have to double check.
Speaker 1
Really? That's a surprise for me, wasn't it like 175 billion?
Helen Byrne
So everyone was there was a kind of debate. Was this a leak? Or was it? Was it a typo? Anyway? It's, it's, well, it seems that it's just been very well distilled from the 175 or whatever. . So I think they...
Speaker 1
But then, that's different. If it's distillation, then you have to first train, right?
Helen Byrne
Yeah, I think they're starting with a larger model. But the models that are actually being deployed, are seemingly getting smaller, or at least plateauing. Because it's just for now, I guess compute costs. It's it's unattainable to deploy a trillion parameter... hundreds of billion parameter model.
Speaker 1
But it's not only that, it's also latency, reliability and whatnot. I wonder whether you can get squeezed out the same performance, like you have these MPTs 30 billion, right. But you kind of train from scratch in that, at that size would be interesting. If someone could kind of compare, or have the ability to compare, like, training from scratch the model of same size, how much does it differ from the distilled model?
Helen Byrne
Yeah, I think unfortunately, at the moment, there is a difference, the hugely over-parameterized model somehow manages to distill down into a much more efficient, smaller, more powerful model than if you train it from scratch. And that was kind of the sort of part of the kind of incentive for the the lottery tickets, those papers that were years ago of, well, if you if you can prune, so it's different to distill. But if you can prune the model down and still get the same performance at inference, without eliminating all of these weights, for a much smaller model, can you train it from scratch? As a sort of model, but it never really, it never really took off. It never really worked properly, as...
Speaker 1
So was the conclusion of that paper that you can't do that?
Helen Byrne
Well, the conclusion of the paper was, hopefully we can, there was a number of follow up papers where they tried. And I think they managed in certain situations, certain settings. But ultimately, it's it didn't really work out as a idea. But yeah, he's one of the the lead author on that paper is the was the chief, or is the chief scientist at Mosaic.
Danijela Horak
I keep hearing about that paper, but I've never actually, really,
Helen Byrne
I mean, I think it was very interesting, four years ago, whenever it came out, it was the best, it was the best paper at one of the big conferences. I think it was ICLR. It was super interesting. But I think, yeah.
Danijela Horak
And it's also like, I have issue with these papers. At the moment, it's like, a couple of years ago, it used to be - and this is just my observation - it used to be like to publish the paper at the top conference, you actually have to include some maths and people didn't like it, people hated it. But nowadays, with these large language models, like you run a set of experiments that are very constrained, like on a very narrow space, and then you're basically saying, Well, this is my conclusion, right? And it's almost like, similar to this parable about the blind man with an elephant and then you just catch one, one part of an elephant to say, hey, you know, the elephant is long and thin, right? And then we all kind of jump on it, because many of us don't actually have the possibilities to run the experiments of that size. So without any proper hypothesis without any proper theory, you're just bound like to sit there and read what's coming in. Many, many many of these experiments are very narrow in their domain, they are not very comprehensive and then you may end up thinking like that this is true for all the possible cases or that it kind of is a general rule, when actually it's not.
Helen Byrne
I think that's the case for, unfortunately, for a lot of these papers. I do feel like more people are speaking up actually, and and trying to change this to get more robust experiments, and maybe some theory.
Danijela Horak
Yeah, but it's not even that. I think what we're missing is like, we're kind of doing it all wrong. I'm not like, I don't criticize, but I'm just the saying what I've seen what I've observed. So for example, in other sciences, like you have a certain model, you have a certain hypothesis that you want to test. There is something that you're testing, you just don't go off and do experiments, like, it's almost like we are reducing AI, to a level of like social sciences almost like to the way how psychologists and social scientists and nutritionists are doing science, right, like they go off, they do tests, they kind of see a bit of a lot of correlations, and then they draw a conclusion. Whereas like, we should look up to physicists who actually have a model, have a theory, then they say, well, if this theory is true, then let's calculate some consequences and say, Okay, well, if this theory is true, and then these consequences have to be true, then we have to go out and do experiments. And the experiments support these consequences, then it means that the theory is probably true. So I don't know, I just find it that we're still still early on in this field.
Helen Byrne
So I know that recommenders isn't within your team really anymore, because it's more on the product side now. But I think it's quite an interesting area. It's quite a well established area of AI. And it's where people that interact with the BBC, will be interacting with the recommender systems. So with iPlayer, or BBC Sounds, I'm sure you have lots of media platforms where people interact with recommender systems. So is there anything you could tell us about what what these models look like? Or the data that's used? So is it users watchings shows, interacting? Is that how you predict what they should watch next?
Yeah, I think it's very interesting how BBC approaches their recommender systems. So we have, we have the one in iPlayer, Sounds and News at the moment. And we are basically not allowed to use any socio-demographic data, any location data, so nothing apart from the user behavior data, and only on the basis of your past behavior on the platform, you can kind of infer and give recommendations for, for what to watch going forward. But I think even that is kind of subject to some kind of business rules and editorial rules that need to be followed. So I think, yeah, at the moment, you know, what you can achieve using that methodology, I think it is limited in a way. But I think that this field, the recommender systems field is bound to be transformed in a major way, you know, by the large language models. And I think not many people out there are actually aware, but it's, you know, it's going to completely transform how we how we approach building some of these algorithms.
In what ways are LLMs being used or starting to be used or potentially used with recommendation systems?
It's just my thought, like, we are obviously using traditional algorithms and are not and as you said, not in my team anymore, but from the research point of view on what I see out there, what others are doing, like in commercial... so I thought that the partnership between Klarna and OpenAI has been an interesting one. So basically, like they are going to offer user experience in the future where you could just go log into their platform and, you know, search for a product. Like through natural language, you know, for example, like my it's my 16 year old son's birthday next week... he likes, you know, football, sports and doesn't like fashion and this can you recommend something under £40 that deliverable tomorrow? I don't know something like that. And that's, that's obviously going to disrupt ecommerce for sure, in a major way. And then you can kind of iterate through it, you know, you get a couple of products, and then you say, okay, you know, well, maybe not like this is just the, you know, he doesn't like this stuff, focus more on something else and whatnot. But the thing is coming for recommenders, right, like, you will log into Netflix, you know, like, you are not any more a passive consumer of what is served to you, you're not any more like an agent that can input like, only one key word to find the piece of content that, that that they want to watch, like, you can actually say what you want to watch, like, I feel like watching period drama this evening, with elements of this and that. And this is just like one way, but there are like thousands of other ways that this technology can be used. And then then of course, the question is, whether you need to have your content in the weights of your LLM, or you can use it in a RAG type of way, this type of kind of parsing. So I think it's super exciting. It would be interesting to see, you know, how the big players in the recommender systems space, like, I think Netflix has long been recognized as the leader and having the best algorithms. So let's, let's see what they come up with, you know, it's definitely going to be like, exciting couple of years in this space.
Yeah, it's interesting. So there's the recommendation of media, which is obviously BBC, Netflix, and then it feels to me like a slightly different kind of side of recommenders, which is the e-commerce side. So I know that Meta, when they published their DLRM paper, they said, and I remember speaking to one of their researchers about this, they said that recommenders is so under published about, relative to the commercial application value of this area, because people are, organizations are developing their own systems, and they want to keep them proprietary, because they don't want to give away their secret sauce to others. But it sort of feels to me like the media side is different, like the media, I guess, with media recommendation, it's probably not as huge a commercial kind of implication on your business. So for Meta or for Amazon, I think that getting the recommendation right and wrong, or whatever can can affect very much the kind of revenue, it has a very direct effect on revenue, and people buy, whether they're going to buy their products. I guess with media, it's it certainly does have an impact and you want to people to watch for longer and listen for longer, but it's slightly less important maybe to your to your business structure?
Danijela Horak
Well, I would agree with you, but maybe kind of add a couple of other reasons why I think that's, that's true. If you're a user logged into a platform, and you're kind of browsing through the catalog, I think there is a certain window that is much larger, within which you're supposed to recommend, not to lose that customer, not to lose that audience member, you know, after a certain point, they just give up and say, okay, there is nothing here to watch for me, I'm giving up. So this window is much larger, like you are allowed to use maybe slightly crappier algorithms. And, and I also a lot, a lot has to do with the content quality, right? So you're not only a platform, serving someone else's content and relying on other people's creativity, like Twitter, or Facebook or Instagram, you basically also have to commission content and serve it to people. So a lot of it is about like editorial decisions as well. So it's very difficult to kind of disentangle how much value is brought on the table by the recommenders and how much is it UX like UX UI? How much is it content and whatnot. But definitely, I think, you know, at one point, people do kind of give up if you don't show them content items that might be interesting to them within that time window.
Helen Byrne
So moving on from the sort of specific domains of the various sort of applications that you use AI for the BBC. A big topic of conversation at the moment is around responsible AI, including sort of explainability, transparency of the models that you're using, I guess working at a publicly funded company like the BBC, this is probably even more important. Would you say that's true? And could you tell us it If If you have any examples of kind of what you have to do at the BBC in terms of using AI and ensuring it's responsible, do you have, do you collaborate with other team members, for example? I don't know, non ML specialists? Or do you have to think about alignment of any model development? Anything along those lines?
Danijela Horak
Absolutely. I think even the BBC had the first their responsible AI and data team before it had data science teams. Of course, I'm I'm kidding. But we were very early on, on that journey. And that's, that's simply because we have such strict editorial guidelines, which permeate the whole, the whole digital product portfolio, and everything needs to be in accordance with these guidelines. The same holds true for the technology we deploy as well. So we're very concerned with bias, you know, what we see now with the larger language model is that, basically, there is a political bias that is present in some of these models. And I think what one of the papers that came out in the ACL this year was saying is that, well, if a certain type of political bias is in the way, it's in the pre-training stage, it's very difficult to kind of get rid of it later on. So, so again, that was like one set of experiments, you know, that paper has come out with that conclusion. But it's, it's an interesting kind of data point to keep in mind. Not to mention other other biases that can be you know, can be seen in the, in some of these models, especially recommender systems, you don't want to kind of put people in echo chambers, you don't want to play this kind of attention economy game. So for us, it's front and center, we actually have a very large, responsible AI and data team, that is comparable to the size of our data teams. It's very, very significant. And they've developed like a set of guidelines and rules, they call them MLEP, machine learning engine principles. And they were published in I think, 2018, or 19. So basically, in theory, every model that is produced, it's almost like a checklist, you have to go through this checklist and say, Is there a gender bias? Is there this bias is there. So a lot of these kinds of questions that have been collected that we have to make sure that they are answered.
Helen Byrne
Yeah, yeah. Interesting. I remember the one of the things that came out the Llama, the Llama paper, when they released Llama v2, which was, I don't know, I can't remember how many pages it was, I didn't read the whole thing. I think it was 80 plus pages. But they really they separated looking at, I'm gonna get this wrong now, but trust, like, kind of correctness shall we say. I can't remember what it was called, and safety. Interestingly, these two kind of objectives can be very conflicting with each other. So yeah, I think it's a really interesting area of research and development.
Danijela Horak
But isn't it like helpfulness and safety, right? Yeah. If you want to have a safe model, then you have to decline to follow certain instructions and the more you decline, then the model is more incentivized to decline things that it's should actually do. So it's a very difficult...
Helen Byrne
It's an interesting open question. Yeah,
Danijela Horak
I think reading Mistral paper, in it they kind of criticize, although they have a very, like they only have like a self check in their model. So, the only safety guidelines they have is like they give it back to the model and ask like whether I should answer this question or not. And they claim that they are model is almost as equally safe as as Llama and it doesn't kind of make, it doesn't decline to follow through to do certain instructions that may be you know, plausible, like how to kill a Linux process. This would be answered by Mistral model but Lama would decline basically, although guys from Meta say that this has been debunked, but what we found is the definitely Llama models don't have the full understanding of certain words when used in in certain contexts. And whether it's like because like they over engineer their safety or whether it's the models are small, to be able to recognize what this word may mean in this context. I don't know really, but it's, it's an interesting, I think debate to be had.
Helen Byrne
I could probably chat to you for two hours. Which is great. I guess maybe just to finish up, I'd love to know, what are you really excited... So if you take your BBC hat off, and just your AI enthusiast hat, what are you sort of most interested in and excited about, whether it's in research or news or whatever. Is there anything?
Danijela Horak
Yeah, well, definitely. I think, like how these language models are going to transform the whole digital ecosystem, I think that's going to be interesting to kind of see how it unfolds for sure. I'm also kind of curious to see what's going to happen to this kind of debate and what's going to happen to our field actually, that's becoming kind of increasingly politicized, the existential risk debate. And it was quite kind of almost sobering to see how all of this kind of unfolds in the public discourse, because what we've seen is that, like, the way how, at first, I've really enjoyed watching some of these debates, like between doomers and tech optimists. But what I think was missing is that they've been conducted in a completely unscientific manner. So like, you would get a debate where you get two people to debate whether these models present existential risk, but also at the same time, no one actually, they have in their minds, different definitions, what existential risk means and they are talking past each other. So this is the first one. So I think like, we could have extracted so much more value from it, I'm super excited that the people from our field are kind of starting to think more philosophically, I think it's great, I think it's like, it's almost like a characteristic of any field that is at the forefront. It's like we had philosophers of the 18th 19th century, being mathematicians then moved into 20th to being physicists, and now it's like the engineers or machine learning engineers. So I think it's a natural progression of things. But what I'd like to see is kind of some more structure. Can we includine a little bit more of that rigor in these debates? I don't know. You know, of course, excited as the next person about what's OpenAI gonna launch next year, next month? You know, let's see where it goes next. You know, it's equally exciting and frightening at the same time.
Helen Byrne
Yup. I know exactly what you mean. Totally agree. Oh, thank you so much Danijela. It's been so great to chat to you and hear your insights and your opinions and to hear about what you're up to at the BBC. So thank you. I could talk to you for hours. I hope I run into you again soon and we can catch up more.
Danijela Horak
Definitely. Thankyou, Helen. Such a pleasure to be a guest on your podcast. Good luck with it. I'm sure you're gonna do brilliantly.
Helen Byrne
Thanks to Danijela, I loved hearing about her experiences at the BBC. If you enjoyed listening, then please leave us a review and subscribe to the podcast. You can also watch us on YouTube. We are @distillationpod on all the main social media channels. So go follow us there if you want to find information on upcoming episodes. I hope you'll join us again soon.